New computational approach uses diagnostic codes and previous doctor’s visits to predict diagnosis of autism spectrum disorder in children
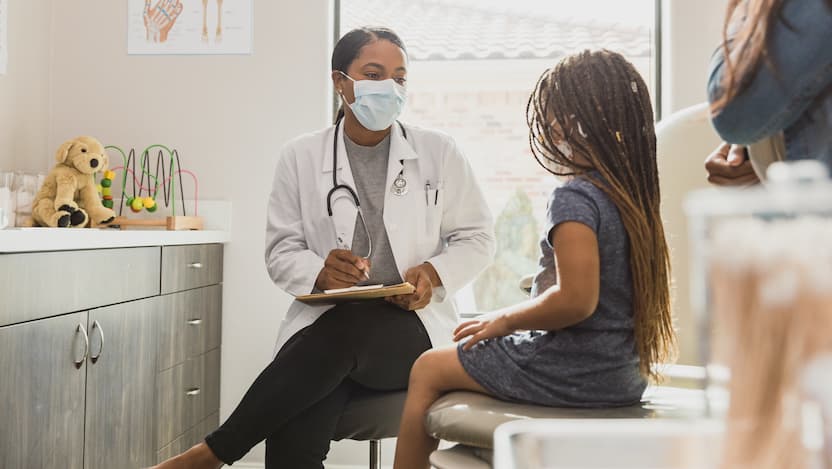
Researchers at the University of Chicago have developed a novel computational approach that can reliably predict an eventual diagnosis of autism spectrum disorder (ASD) in young children, without the need for additional blood work or procedures, using only diagnostic codes from past doctor’s visits. The new approach reportedly reduces the number of false positive ASD diagnoses produced by traditional screening methods by half.
ASD can be diagnosed as early as age 2, but false positives flagged by the initial screens traditionally used today can delay the confirmation of a true diagnosis. Given the value of early intervention and the limited number of trained professionals, tools that can potentially reduce the pool of patients required to undergo the lengthy, multistep process to receive an official positive diagnosis can have a profound impact on patient care.
Traditionally, questionnaire-based screening tools are used as the first step in identification of ASD. “But, these are prone to inaccuracies that may arise due to things such as a language barrier or culture barrier, and can give rise to inaccurate diagnoses. By only looking at the data as objectively as possible, our approach avoids some of the pitfalls of traditional screening approaches,” said lead author Dmytro Onishchenko, a senior scientist in the Zero Knowledge Discovery (ZeD) Lab led by Ishanu Chattopadhyay, PhD, Professor of Medicine.
The study, a collaboration between the ZeD Lab and University of Chicago developmental pediatricians Dr. Michael E. Msall, MD, and Dr. Peter J. Smith, MD, was published on October 6 in Science Advances.
Using only sequences of ICD9 and ICD10 (International Classification of Diseases) diagnostic codes generated from past doctor’s visits, which are available for any consenting patient, the researchers were able to leverage known comorbidities of ASD to reliably predict an eventual positive diagnosis.
“Using the information already being gathered and being able to harness it for this kind of exploration and clinical use is exciting, and it really has the potential to be a game changer,” said Smith.
The researchers’ new algorithm determines an autism comorbid risk score (ACoR), which estimates the risk that a child with a given timeline of diagnoses will eventually receive a confirmed ASD diagnosis. The research applied advances in medical informatics to over 30 million de-identified diagnostic sequences representing over 15,000 distinct ICD codes, originating from the Truven Health Analytics and University of Chicago Medical Center (UCM) databases. The team separated these profiles into positive (i.e., an official ASD diagnosis) and controls. They then applied algorithms that “learned” patterns representative of ASD-positive cohorts compared to ASD-negative cohorts. This research strategy allowed them to find which disease categories contribute to the ACoR and how much each category contributes.
When these risk scores are calculated for an individual patient, the researchers can quantify how far their unique diagnostic timeline deviates from either the positive or control group. When that risk score crosses a threshold, a patient can be flagged as possibly requiring interventions.
By several standard metrics, ACoR outperformed the commonly used questionnaire-based M-CHAT/F screening method, as well as other methods that have made use of comorbidity patterns, including a higher probability that a flagged patient will receive a confirmed ASD diagnosis. Importantly, the researchers were able to flag patients as at-risk more than one year earlier than their actual diagnosis.
Importantly, ACoR performed consistently well for different racial and ethnic groups and even in U.S. counties where diagnostic resources are scarce. “A lot of what we have done is take the data and processes available in better connected systems and apply them to less well supported healthcare communities. We know for instance that African American individuals are frequently diagnosed later, which has an impact on long term care. This type of technology could overcome some of these structural barriers,” said Smith.
None of this should be taken to mean that the old paradigm for autism screening should be thrown out. Despite their exciting results, the researchers see this new tool as complementary to methods like M-CHAT/F.
“Many machine learning-based approaches, particularly those trying to do autism screening, may aim to replace or mimic the physician. But people like Dr. Msall and Dr. Smith have been doing this for many years. Maybe someday machine learning will be able to replace human decision making, but we are not there yet,” said Chattopadhyay. “The point of this approach is to leverage underutilized modalities, to do computations that the human brain cannot, to complement the physician.”
In addition, Msall said, “Our goal is to perform enablement diagnostic confirmation and help families access the pathways that promote on-going communicative, social and adaptive learning during times of maximum neuroplasticity.”
In fact, when used in tandem with the screening results from M-CHAT/F, ACoR performs even better. There is no substitute for the expertise of a trained specialist or the careful observation of parents, but when 85% of the patients flagged by M-CHAT/F are false positives, objective, data-driven approaches like ACoR, which can be administered even without the patient being present, can fill in the gaps sometimes created by the subjectivity of other measures. The ZeD lab hopes to see their tool widely adopted so that it can reduce the number of false positive patients, who would still need to undergo subsequent screening, and cut down the waiting times for families seeking care for their children.
“Our paradigm shift is more than appropriate early identification. Our paradigm shift is engagement, community flexibility, and recognition of neurodiversity,” said Msall. “Autism is an epidemic, but children can still thrive with this diagnosis. We can bring systematic population-level science, data science, and decision-making science together so that we can appropriately support the families in new and creative ways.”
The study, “Reduced false positives in autism screening via digital biomarkers inferred from deep comorbidity patterns”, was supported by the Defense Advanced Research Projects Agency (DARPA) project number HR00111890043/P00004. Additional authors include Yi Huang and James van Horne, both of the University of Chicago.