Using math to understand how tiny molecules regulate gene expression
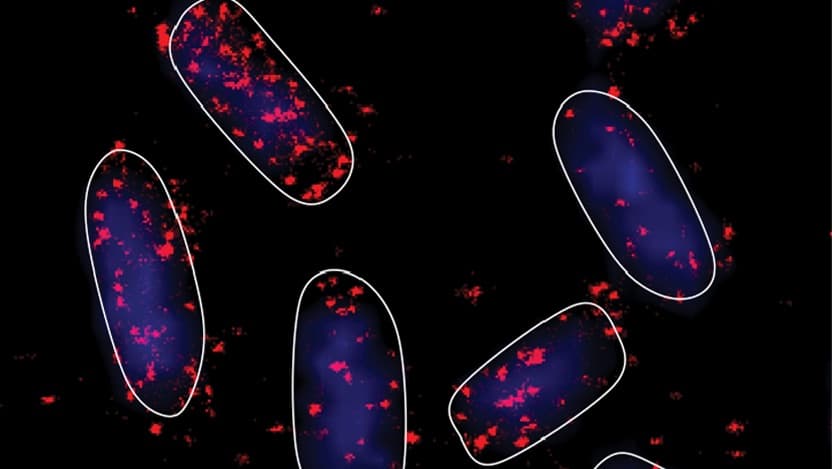
Existence is difficult, especially in stressful environments. That may seem like a metaphysical statement, but it’s true for all living creatures — even the microscopic ones. Consider the lowly bacterium. Without stress coping mechanisms that humans use like meditation and journaling, bacteria must rely on nature’s original form of stress management: gene regulation.
Gene expression describes the process by which DNA gets transcribed into RNA, and then RNA is subsequently translated into proteins. Gene expression is therefore critical for many activities such as cellular movement and nutrient transportation. All cells are constantly expressing genes, but sometimes the details of that expression may change, which can determine whether or not a cell survives. For example, E. coli produce a certain number of transporter proteins to consume sugar under ordinary circumstances, but in a stressful environment, that amount may be too many or too few. Rather than producing the normal number of transporter proteins, dooming the cell to an unfortunate demise, bacteria can use molecules called small RNAs, or sRNAs, to modulate the number of proteins to survive in its current environment.
sRNAs are a type of RNA that are short, highly structured, and usually not translated into proteins. Instead, they regulate the expression of other genes. They typically do this by preventing messenger RNAs (mRNAs) from being translated into proteins. But sRNAs also regulate some mRNAs more effectively than others, and it’s possible that those differences could play a role in how cells respond to stress efficiently.
In the lab of Jingyi Fei at the University of Chicago, I study how bacteria use those sRNAs to regulate their gene expression. We’re interested in how the underlying kinetics, or the rates at which different regulatory steps (degradation, binding, etc.), dictate the regulatory hierarchy of an sRNA. Understanding how bacteria prioritize sRNA-mediated gene regulation will help us clarify important details about how sRNAs function, which could in turn allow us to use sRNAs to benefit human health.
We study an sRNA called SgrS and three of its known regulatory targets, mRNAs called ptsG, manX and purR. In E. coli, SgrS responds to glucose-phosphate stress, a type of stress characterized by an overaccumulation of phosphorylated sugars within the cell. ptsG, manX and purR are among the many genes involved in transporting sugar phosphates into E. coli. We use mathematical modeling of SgrS-mediated regulation to show how the numbers of sRNAs, mRNAs and proteins change over time according to specific kinetic rates.
Because the reactions involved in the regulatory process occur too fast and with molecules too small to measure directly, we instead computationally determine the most likely values for the kinetic parameters by fitting the model to real data. In a recent study, that data came in the form of microscope images of mRNAs, sRNAs and proteins that showed how the number of each molecule changed over time after we exposed cells to glucose-phosphate stress. By using a technique called Fluorescence In Situ Hybridization (FISH), we were able to specifically visualize how just one of the mRNA targets responded to SgrS. Doing this for ptsG, manX and purR individually, we were able to determine the kinetics of SgrS regulating each mRNA.
We found that the rate at which SgrS was able to find and bind the target mRNA lined up exactly with the known order of the regulation hierarchy. That rate was forty times higher for ptsG, which is known as the highest priority target of SgrS, than it was for purR, a known lower priority target. These results help clarify observations that had previously confounded biochemists, explaining the mechanisms behind the regulation hierarchy.
In addition to what we learned about the kinetic determinants of sRNA-mediated regulation, we also unexpectedly learned something about the timing of the regulation. Throughout the history of the study of sRNAs, they have typically been described as post-transcriptional regulators, meaning that they begin to regulate their target mRNAs only once the mRNAs are fully synthesized. However, when we tried to fit a model with the post-transcriptional regulation assumption to our data, the numbers didn’t work.
Looking for answers, we turned back to the model and asked what could be wrong. We guessed that SgrS wasn’t waiting for its target to be fully synthesized, but instead started regulating it while it was still being transcribed. In other words, we hypothesized that SgrS was a co-transcriptional regulator, not just a post-transcriptional regulator. After experimentally proving that SgrS had the capability to do so, we returned to the computers and found that by building co-transcriptional regulation into the model, we were now able to replicate the data. We later proved that the same model could successfully represent an entirely separate sRNA regulatory network. Therefore, co-transcriptional regulation might be more widely used by sRNAs than previously thought.
Using microscopes, computers and math, we were able to better understand the physical mechanisms of sRNA-mediated gene regulation. This is exciting because the physics likely translate to other systems, such as different sRNA-mRNA combos, or even to cells other than E. coli. We hope that a more basic understanding of the sRNA regulatory process will someday allow translational scientists to use sRNAs to benefit human health.